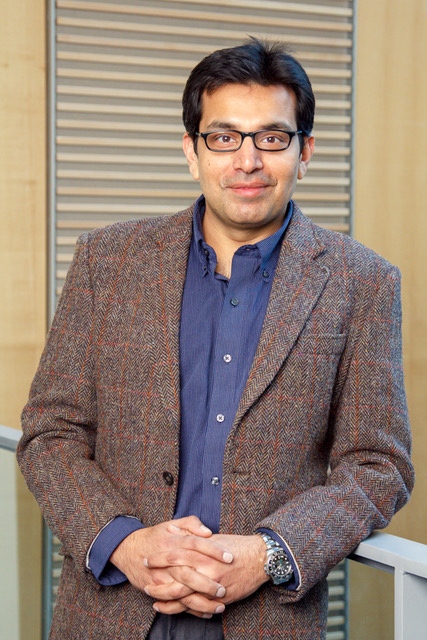
Faisal Qureshi
PhD
Professor
Faculty of Science
Exploring theory and models for the next generation of ad hoc networks of smart cameras providing perceptive coverage of extended spaces
Contact information
Science Building - Room UA4071
North Oshawa
2000 Simcoe Street North
Oshawa, ON L1G 0C5
905.721.8668 ext. 3626
faisal.qureshi@ontariotechu.ca
http://vclab.science.ontariotechu/
Research topics
- Visual Sensor Networks
- Multicamera Tracking
- Graphics for Vision
- Video Summarization
- Stereo Depth Estimation
- Optical Flow Estimation
- Hyperspectral Image Analysis
- Deep Networks for Computer Vision
- Activity Analysis
- Action Recognition
- Image Inpainting
- Video Surveillance
Areas of expertise
- Computer Science
- Computer Vision
- Deep Learning
- Camera Networks
- Video Summarization
- Graphics for Vision
Background
Dr. Qureshi received the B.Sc. degree in Mathematics and Physics from Punjab University, Lahore, Pakistan, in 1993, the M.Sc. degree in Electronics from Quaid-e- Azam University, Islamabad, Pakistan, in 1995, and the M.Sc. and Ph.D. degrees in Computer Science from the University of Toronto, Toronto, Canada, in 2000 and 2007, respectively. He is a Professor of Computer Science in the Faculty of Science, Ontario Tech University, where he leads the Visual Computing lab. His research focuses on computer vision, and his scientific and engineering interests center on the study of computational models of visual perception to support autonomous, purposeful behavior in the context of camera networks and self-organizing visual sensor networks. Dr. Qureshi is also a Guest Professor, at Mid Sweden University, Sweden.
Education
- PhD Computer Science University of Toronto 2007
- MSc Computer Science University of Toronto 2001
- MSc Electronics Quaid-e-Azam University 1995
- BSc Mathematics and Physics (Minor) Punjab University 1992
Courses taught
- CSCI 1061U - Programming Workshop II
- CSCI 2050U - Computer Architecture
- CSCI 3050U - Computer Architecture II
- CSCI 3070U - Design and Analysis of Algorithms
- CSCI 3010U - Simulation and Modeling
- CSCI 3090U - Computer Graphics
- CSCI 4110U - Advanced Computer Graphics
- CSCI 4220U - Computer Vision
- Graduate courses in Computer Vision, Deep Learning, and Machine Learning
Involvement
-
Selected publications
- “A Residual-Dyad Encoder Discriminator Network for Remote Sensing Image Matching,” Khurshid, N.; Mohbat; Taj, M.; and Qureshi, F. IEEE Transactions on Geoscience and Remote Sensing, 14pp., 2019. In press
- “Joint Spatial and Layer Attention for Convolutional Networks,” Joseph, T.; Derpanis, K.; and Qureshi, F. In 30th British Machine Vision Conference (BMVC19), 14pp, Cardiff, Sep 2019.
- “Neural Networks Trained to Solve Differential Equations Learn General Representations,” Magill, M.; Qureshi, F.; and de Haan, H. In The Thirty-second Annual Conference on Neural Information Processing Systems (NuerIPS 18), 11pp, Montreal, Dec 2018.
- “Fast Estimation of Large Displacement Optical Flow Using Dominant Motion Patterns & Sub-Volume PatchMatch Filtering,” Helala, M.; and Qureshi, F. In 14th Conference on Computer and Robot Vision (CRV 17), 8pp, Edmonton, May 2017. Best Computer Vision Paper
- “Stereo Reconstruction of Droplet Flight Trajectories,” L.A. Zarrabitia; F.Z. Qureshi; and D.A. Aruliah. IEEE Transactions on Pattern Analysis and Machine Intelligence, 27(4), April, 2015, 847–861.
- “Smart Camera Networks in Virtual Reality,” F.Z. Qureshi; and D. Terzopoulos. Proceedings of the IEEE, 96(10), October, 2008, 1640–1656, (Special Issue on “Smart Cameras”).
- “Intelligent Perception and Control for Space Robotics: Autonomous Satellite Rendezvous and Docking,” F.Z. Qureshi; and D. Terzopoulos. Journal of Machine Vision Applications, 19(3), February, 2008, 141–161.